Using X-rays to look inside the cell
Issue: Imaging
13 February 2018 article
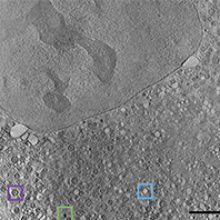
Protein misfolding disorders are a class of diseases associated with unchecked protein misfolding and aggregation. The two examples we focus on are Huntington’s disease and Alzheimer’s disease, which are both neurodegenerative, protein misfolding disorders and share common features.
Huntington’s disease (HD) and Alzheimer’s disease (AD) are disorders of the brain in which neuronal cells die, and are strongly associated with the aggregation of different proteins into amyloid fibrils (Fig. 1). In HD, mutations in the huntingtin gene lead to the production of huntingtin protein consisting of abnormally long stretches of the amino acid glutamine. There is a relationship between the length of the polyglutamine repeat and clinical onset; the longer the repeat, the earlier in life the disease will manifest.
Fig. 1. The process of protein misfolding. Monomeric proteins or peptides can, under certain conditions, undergo conformational changes that lead to a higher likelihood of further aggregation. At the dimer or oligomer stages, a critical nucleus is formed that recruits additional monomers, leading to formation of protofibrils and eventually amyloid fibrils of the type observed in HD and AD brains.
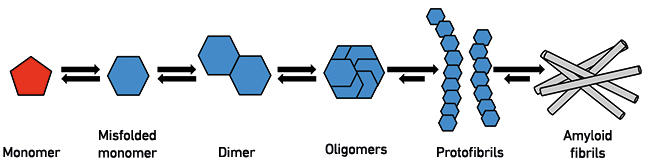
Protein misfolding in disease
AD is associated with the misfolding of two proteins, amyloid-β and tau. Both are able to self-assemble; however, they have very different structures and functions. Amyloid-β (Aβ) is a relatively short (39–42 amino acids) peptide with no established function and exists in an unfolded, disordered conformation. Tau is a much larger protein and is known to be important for microtubule stabilisation. In AD brains, Aβ fibrillar aggregates are found in the extracellular space whereas tau aggregates are deposited intracellularly. Many lines of evidence suggest that Aβ misfolding is the trigger of AD that leads to downstream effects, including tau aggregation, synapse loss, and eventual neuronal cell death.
Although HD and AD have many similarities, they manifest differently, with HD being primarily a movement disorder and AD affecting memory. Although much is known about protein misfolding disorders (PMDs), precisely how protein misfolding leads to neuronal dysfunction and loss remains a pressing question in neurodegeneration research.
In both AD and HD, a number of systems are impaired in response to mutant huntingtin or misfolded Aβ including protein folding and degradation pathways, synaptic signalling, and mitochondrial function. Whether these dysfunctional effects of misfolded proteins are direct or indirect, which aggregated forms are the most toxic, and how the disturbances to different cellular functions interplay with or exacerbate each other is not clear at present.
Viewing the inside of the cell
Many of the functional disturbances occur in parts of the cellular machinery that can be visualised using various microscopy techniques, such as fluorescence light microscopy, resin-embedded serial-sectioning scanning electron microscopy (SEM) or transmission electron microscopy (TEM), and here cryo soft X-ray tomography. Mitochondria, lysosomes, nuclei, autophagosomes and synapses are all clearly identifiable subcellular compartments that may show structural alterations, providing enormous benefit to our understanding of disease pathogenesis. For example, autophagic vacuoles and lysosomes accumulate in neurons in AD brains, suggesting damage to the protein quality control network. Similarly, morphological changes both to organelles associated with protein degradation and mitochondria have been observed in HD. These observations can give clues as to how diseases are caused, the effects of protein misfolding on cells and the underlying molecular mechanisms of neurotoxicity.
A structural understanding of PMDs can provide functional context across multiple levels. Cryogenic electron tomography (CryoET) can be used to provide a limited cellular context of thin areas such as the edges of cells or the processes of neurons. The cells can also be processed to an appropriate thickness using cryo-sectioning or cryo-focused ion beam milling techniques. However, each of these techniques provide data of only limited areas of the cell. Cryogenic soft X-ray tomography (cryoSXT) alleviates this concern, but at the expense of resolution.
Fig. 2. CryoSXT of an intact mammalian cell expressing mutant huntingtin. Various organelles (lipid droplets, green box; empty vesicles, blue box; mitochondria, purple box) are identifiable due to their inherent characteristics. Bar, 5 μm. |
![]() |
CryoSXT uses X-rays to visualise vitrified cells grown on TEM grids. Tilt series are collected and then reconstructed into a 3D volume representing the cell as it was when frozen. This sample preparation method does not use fixatives, stains or resin. In this way, it is possible to collect data through even the thickest part of the cell, at 30–50 nm resolution, using the inherent contrast of the sample.
The power of X-rays
One area of interest, studied on Beamline B24 at Diamond Light Source, is the changes to mitochondrial structure and organisation during expression of mutant huntingtin fragments. Using cryoSXT, large regions of the cell, such as the nucleus, nucleolus and cytoplasm, are clearly recognisable (Fig. 2). The cytoplasm in this disease state cell is extremely crowded. Lipid droplets are easily spotted as dark black organelles (green box) and empty vesicles can be spotted as bright white organelles (blue box). Mitochondria can be recognised structurally due to the presence of cristae (purple box), making the study of their dysfunction here relatively straightforward. However, without further labelling, it is difficult to determine the identity of many of the other organelles.
To address this, if desired, fluorescence light microscopy (FLM) images can be acquired, while keeping the sample at cryogenic temperatures. This enables the specific localisation of events or areas of interest (Fig. 3). This was carried out in collaboration with the Serpell Group at the University of Sussex, to examine changes to lysosome structure and organisation during exposure to oligomeric forms of aggregated Aβ. To explore these intracellular changes, neurons were treated with green fluorescently labelled Aβ oligomers (top image), while lysosomes were fluorescently labelled red using LysoTracker (not shown). Cells were imaged using a cryogenic fluorescent microscope to identify the localisation and distribution of oligomers (purple boxes) and lysosomes (not shown) within the cell. Following this, the same cells were imaged using cryoSXT, revealing the cellular architecture at higher resolution. The overlay of fluorescent signals with X-ray data is critical in providing an annotated map of a neuron deteriorating in response to misfolded forms of Aβ.
Fig. 3. Correlative imaging of aggregates. Fluorescently labelled Aβ oligomers within neurons can be observed using cryo-fluorescence microscopy (upper panel), providing limited-resolution localisation information. Imaging the exact same part of the cell usign cryoSXT reveals they are located within membrane-bound vesicular structures (lower panel). Combining these methods gives an annotated cellular map showing how oligomers are distributed within the cell body.
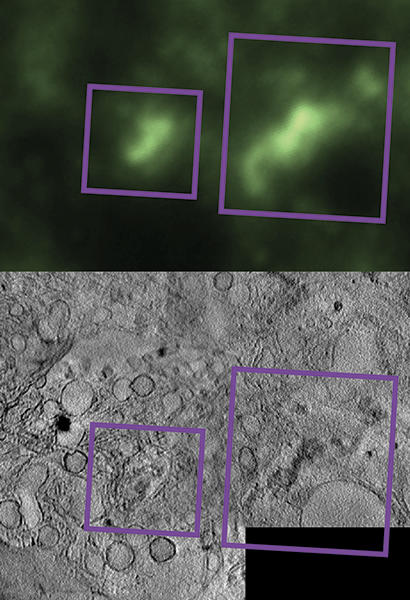
People powered research
The greatest bottleneck in this pipeline is the segmentation and analysis of the data. Segmentation is the process of identifying and annotating areas or objects of interest. The current standard in the field is to trace the outlines of each object of interest, on each slice of the 3D data. This is usually a fully manual process, making it tedious, slow and variable. Recently, the development of a new software algorithm has led to the use of machine learning to reduce the amount of user time spent on this task. The user now provides a small amount of markings for each region of interest, which is then used as training data to teach the algorithms the characteristics of each region so that it can predict a full segmentation. Using these techniques, the time to fully segment a single, standard 3D volume has decreased from approximately one month to one week of person-hours. While this is a major improvement, it is still too slow when considered in the context of the experiment at hand. Multiple cells, from both the disease and wild-type state, should be quantitatively assessed to ensure a relevant biological finding, meaning this type of study would still take months of person-time to generate the segmented volumes. Even with this herculean effort, the output segmented volumes would still represent a single, subjective interpretation of the data.
To begin addressing this bottleneck, we have begun a project called Science Scribbler through Zooniverse, an online platform that uses citizen science to answer research questions. This model will allow us to collect manual input from multiple people to use as training data to create segmentation predictions. This will speed up the process of segmenting data, while also decreasing the subjectivity of the task by querying more people. We have recently tested one of the tasks during a public Inside Diamond Open Day at Diamond Light Source. Interested attendees were asked to make marks in each of the large regions in a 2D image (Fig. 4). From these marks, predictions were calculated for the 100 central slices of the 3D volume. In many cases, the predictions find and follow the boundary between the nucleus and cytoplasm quite well. Using these preliminary data, we can identify an ideal strategy for collecting and using segmentation data from citizen scientists. It will also pave the way for the application of deep learning strategies in the future because large amounts of data can be segmented in this way.
Using a lower resolution, but more forgiving, structural biology technique can provide important information about the structures present in various protein misfolding disorders in vivo, the interactions between these structures and other cellular components, and the overall disease state of the cell. Each technique has positives and negatives associated with it that will impact the sample preparation, imaging parameters, and processing decisions. However, across many imaging techniques, segmentation is the bottleneck, necessitating further development in this area.
Fig. 4. Volunteers mark images to teach an algorithm to segment. In many cases, using these marks, the algorithm was able to correctly segment the two large regions (nucleus and cytoplasm) from each other.
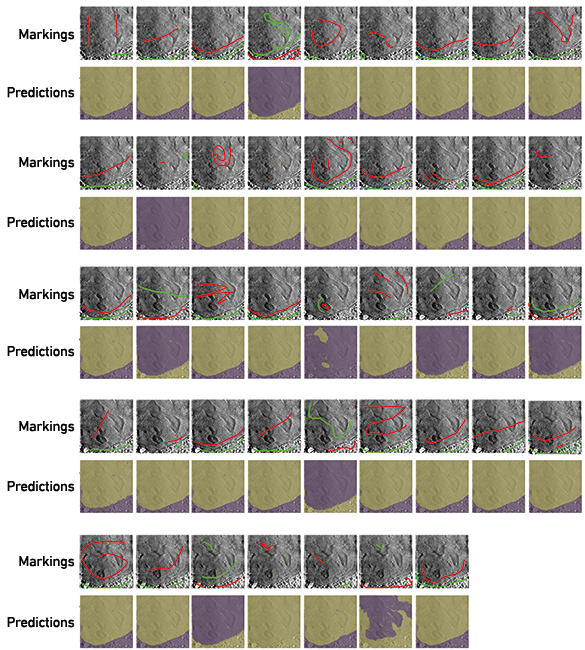
Further reading
Duke, E. & others (2014). Biological applications of cryo-soft X-ray tomography. J Microsc 225, 65–70
Gerakis, Y. & Hetz, C. (2017). Emerging roles of ER stress in the aetiology and pathogenesis of Alzheimer’s Diesease. FEBS Journal, epub ahead of print.
Labbadia, J. & Morimoto, R. I. (2013). Huntington’s disease: underlying molecular mechanisms and emerging concepts. Trends Biochem Sci 38, 378–385.
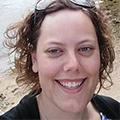
Michele C. Darrow
Life Science Division, Diamond Light Source, Didcot, Oxfordshire OX11 0DE
Michele Darrow is a postdoctoral research associate at Diamond Light Source. She received her doctorate from Baylor College of Medicine in 2015. Her research focuses on correlated imaging techniques and annotation for the purpose of studying interesting biological questions about protein misfolding disorders, and cellular infection and treatment.
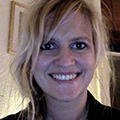
Karen E. Marshall
Biochemistry Department, University of Sussex, Falmer, Brighton BN1 9RH
[email protected]
Twitter: @KarenEMarshall
Karen Marshall is a postdoctoral researcher at the University of Sussex. She has a background in amyloid structure and protein misfolding and is currently interested in how misfolded proteins cause detrimental effects to cellular ultrastructure.
What skills are required in your position on a day-to-day basis?
Michele: Research science is very collaborative so time management and communication are important skills. In addition to the knowledge and skills gained from the study of your field of science, a questioning and imaginative mind, and willingness to discuss, debate and change your mind based on evidence are all useful.
Karen: Skills range from the technical – diligence, precision, a steady hand and good planning – to making sure experiments run smoothly. Good social and communication skills are crucial as research is collaborative and interactive.
What advice would you give to someone starting out in this field?
Michele: A good work-life balance is essential. Burnout and bullying are real problems in research science. You will have to be assertive and take steps to ensure your own happiness.
Karen: Make sure you are determined and have (or can develop) a thick skin. The peer review process can be quite defeating, but if you have a genuine passion and curiosity for your subject, research is a very rewarding job.
Images: Fig. 1. Karen Marshall. Fig. 2. Michele Darrow. Fig. 3. Matthew Spink from Diamond Light Source. Fig. 4. Michele Darrow.