Fitness compensation in M. tuberculosis: A game of genetic chess
Posted on February 21, 2024 by Viktoria Brunner
Viktoria Brunner takes us behind the scenes of their latest publication 'Compensatory mutations are associated with increased in vitro growth in resistant clinical samples of Mycobacterium tuberculosis' published in Microbial Genomics.
”If we use antibiotics when not needed, we may not have them when they are most needed.” - Tom Frieden, CDC
This statement by the former director of the Centers for Disease Control and Prevention is now more relevant than ever. Administering antibiotics inappropriately can further the spread of antimicrobial resistance (AMR), hence diagnostics are just as important as the discovery of new drugs for bacterial disease treatment. This is why the investigation and prediction of antibiotic resistance are the main focus of my PhD.
Hi, I am Viktoria Brunner, a Computational Biology PhD student working at the Nuffield Department of Medicine at the University of Oxford. I am part of the Modernising Medical Microbiology unit based at the John Radcliffe hospital. Our group aims to leverage genetic and structural data to improve resistance prediction and hence the diagnostic workflows in the clinics. In doing so, we hope to make the process of determining which antibiotics should be administered to each patient much faster and more reliable. But we also use the clinical data we have at hand to get insights into the genetic determinants underlying antibiotic resistance and its spread. Our group has access to an incredible wealth of anonymised clinical data. I ended up working with a collection of more than 70,000 M. tuberculosis genomes that had been collected over the course of more than 5 years by the CRyPTIC consortium1.
I’m incredibly lucky to get to work with a dataset of this size, especially in the early stages of a PhD. But sometimes when dealing with these large amounts of data, being a biologist can feel a bit like being an archaeologist: You know that there is information of immense value hidden somewhere underneath, but it is not quite clear how to get to it, since you won’t necessarily know where and how deeply it is buried. The biggest challenge might be to determine what it is you are actually looking for. Fortunately, you can get some idea of where to start looking by considering knowledge that is already available, thereby potentially saving countless hours of exploring.
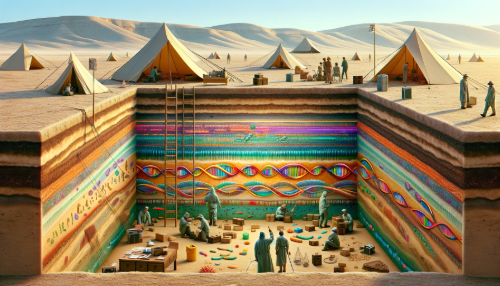
In our case, we were building on knowledge from previous studies of AMR in M. tuberculosis. There are four commonly used antibiotics for the treatment of tuberculosis infections. One of them is the drug rifampicin, which targets the RNA polymerase. In bacteria that are susceptible to the drug, amino acids near the active centre of the RNA polymerase bind to rifampicin. The bound rifampicin stalls protein production and hence causes the bacteria to die. Resistance to this drug mostly arises through mutations near the active site that prevent rifampicin from binding. Since these resistance-conferring mutations introduce changes near the active site of the RNA polymerase, it is not surprising that they also introduce a fitness cost2. In the absence of antibiotics, this makes the bacteria less competitive when compared to susceptible ones.
But much like in a chess game, bacteria with lower fitness can escape their fate of being out-competed by their susceptible counterparts through some clever moves. While resistance mutations might have lowered their competitive fitness, other mutations can arise that compensate for this disadvantage345. And indeed, the low fitness phenotype in M. tuberculosis has been observed to be partially or completely rescued by the presence of so-called compensatory mutations (CMs) in vitro. The existence of CMs gives a plausible explanation for the persistence of resistance long after antibiotic treatment is stopped, when the fitness cost should lead to extinction of the resistant bacteria. This potential makes CMs a very interesting field of study.
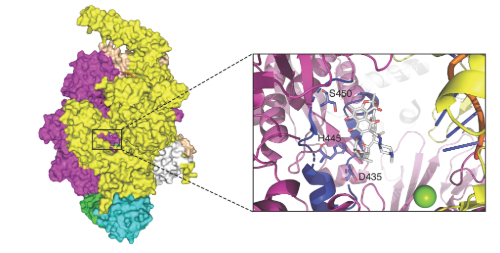
To further our understanding of resistance spread and persistence, it would be useful to construct a comprehensive list of CMs. While many CMs have been characterised already, our dataset has better coverage and statistical power due to its sheer size. It would also be useful to directly observe the effect of the different mutations on the fitness of M. tuberculosis. If you want to learn more about our approach to this problem, just have a look at our latest paper, published in Microbial Genomics. In this publication, we describe how we managed to identify 51 high-confidence CMs through statistical association testing. We also show the negative effect of resistance mutations on in vitro fitness of M. tuberculosis, and the recouping effect of CMs in some lineages of these bacteria.
As introduced in the beginning, AMR is spreading at an alarming pace. The fitness of resistant bacteria plays an important role in this spread, because it affects transmissibility of bacterial infections. Since our results indicate that compensation can raise fitness in resistant populations of M. tuberculosis, it appears to be an important factor in the resistance spread in these bacteria. Strains showing increasing levels of fitness compensation, like the highly virulent Lineage 2, hence deserve special attention in AMR surveillance.
It was a real pleasure to work with the genetic and in vitro growth data resulting from the CRyPTIC project, and I think the application beautifully showed how large amounts of diverse, high-quality data can yield insights into a phenomenon. However, the potential of the dataset is not exhausted, and we are now actively using it as a training dataset for deep learning models able to predict resistance.
To find out more visit
https://www.vbrunner.me or https://fowlerlab.org
References:
[1] The CRyPTIC Consortium (2022) A data compendium associating the genomes of 12,289 Mycobacterium tuberculosis isolates with quantitative resistance phenotypes to 13 antibiotics., PLoS Biology 20:e3001721. https://ftp.ebi.ac.uk/pub/databases/cryptic/release june2022/.
[2] S. Gagneux, C. D. Long, P. M. Small, et al. (2006) The Competitive Cost of Antibiotic Resistance in Mycobacterium tuberculosis, Science 312:1944–1946.
[3] G. Brandis, M. Wrande, L. Liljas, et al. (2012) Fitness-compensatory mutations in rifampicin-resistant RNA polymerase, Molecular Microbiology 85:142–151.
[4] A. K. Alame Emane, X. Guo, H. E. Takiff, et al. (2021) Drug resistance, fitness and compensatory mutations in Mycobacterium tuberculosis, Tuberculosis 129:102091.
[5] T. Song, Y. Park, I. C. Shamputa, et al. (2014) Fitness costs of rifampicin resistance in Mycobacterium tuberculosis are amplified under conditions of nutrient starvation and compensated by mutation in the subunit of RNA polymerase, Molecular Microbiology 91:1106–1119.