Microbiota From the Computational Perspective of a Non-Computer Scientist
Posted on October 16, 2023 by Eric Nayman
Eric Nayman takes us behind the scenes of their latest publication 'Microbiome Depiction Through User-Adapted Bioinformatic Pipelines and Parameters’ published in Journal of Medical Microbiology.
My name is Eric Nayman, and I am currently a 4th year MD Candidate at the Herbert Wertheim College of Medicine at Florida International University (FIU) in Miami, FL. For the last four years, I have been working under the aegis of Professor Kalai Mathee as part of the FIU Bioinformatics Research Group, which focuses on the development of techniques for state-of-the-art multi-omics analysis as they pertain to the human microbiome.
Microbiome science is an exciting and relatively new field in the biomedical sciences. Our resident microbial flora is in constant interaction with our own cells, and this exchange shapes the structure and function of both counterparts. Thus, the microbiome has a significant role in human health and disease. As a future physician and pathologist, I hope to further our understanding of how the microbiome contributes to pathophysiologic processes, and exploit this for the development of novel diagnostics and therapeutics, which is an area of study already underway.
However, studying the microbiome is actually quite challenging because it is difficult to consistently identify how microbiota change and adapt across a wide spectrum of conditions. The work my team recently published in the Journal of Medical Microbiology describes how we sought to ameliorate this insufficiency through bioinformatics and, ultimately, improve how well we can characterize microbiota.
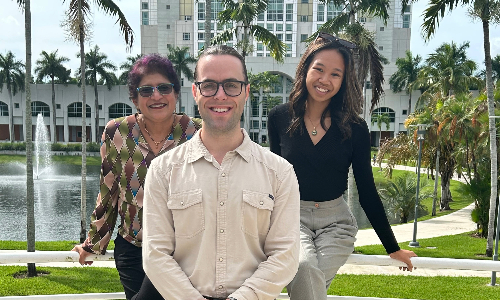
From left to right, Professor Kalai Mathee, Eric Nayman (FIU Medical Student) and Alayna Gumabong (FIU Undergraduate).
For the first part of our project, we had to set up four very different bioinformatic pipelines using the recommendations set forth by the creator of each. Given my biomedical background, this was initially a task far beyond my comfort level. Though, as time went on and through much trial and error, we learned as a team, both data and biological scientists alike. We first performed several trial runs using various genomic datasets to benchmark our workflows. It was through these initial quality control steps that we realized that the crux of our work would address how these well-established but error-prone pipelines are commonly used.
Early on, our workflows discarded up to 80% of the input genomic data, and, as a result, generated largely inaccurate microbial compositions. After we realized this, we tweaked several of the parameters across the workflows and benchmarked these “user-adapted” pipeline runs against how these pipelines are commonly used, which is largely as recommended by their online tutorials. While the tutorials are valuable starting points, they do not account for the uniqueness of each individual user’s dataset. We were able to compare the workflows we adapted to the tutorial-recommended versions because we used a microbial mock community of known composition.
The manipulation of the parameters within each workflow took much official (PubMed and Google Scholar) and unofficial (online forums) literature review. It was through reading what others had tried and demonstrated that we were able to come up with the best versions of our workflows. At the beginning, I distinctly remember the online forums being much richer in information than the published literature. Though, as this project took several years to complete, the literature grew, but I still appreciate how dedicated members of the community were in those corners of the internet.
We hope that our work helps those studying the microbiome to be able to better characterize the composition of their sample. Though, more than that, our work highlights how important it is for biologists and clinically oriented scientists to strive for a deeper understanding of the computational protocols that so significantly impact their work. We demonstrate how striking a few keys on the keyboard can drastically change the outcome of an analysis that holds an increasingly promising clinical potential.